DIC Symposia: 2024
The 6th DIC annual symposia
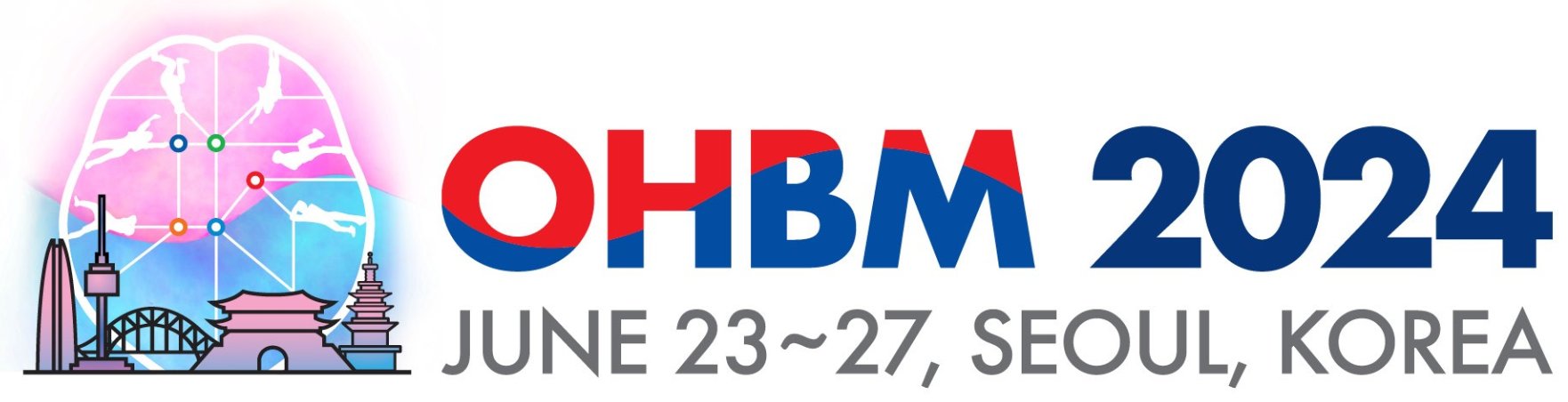
Diversity, Equity, and Inclusivity in Artificial Intelligence and Neuroimaging
Organizers:
Kangjoo Lee, Lucina Q. Uddin, Julia W. Y. Kam, Davynn Tan
Timeliness, importance of topic and desired learning outcomes:
There is an increased recognition that some groups are historically marginalized in ways that ultimately hinder both social and scientific progress. Since the launch of a Diversity and Gender Task Force at OHBM, which aimed to address multiple forms of inequity with respect to gender balance and geographical representation on the Council, a growing body of OHBM initiatives have worked towards tackling a range of issues surrounding underrepresentation at OHBM, including the creation of a Diversity and Inclusivity Committee (DIC). The DIC works to address multiple dimensions of bias, as demonstrated by the success of our inaugural symposium in 2019 focusing on gender biases in academia, the second virtual symposium in 2020 focusing on neuroscience and the LGBTQ community, the third virtual symposium in 2021 on the topic of racial bias in neuroscience, the fourth symposium in 2022 on the Asian perspective on the effects of social, cultural and language barriers on inclusivity at OHBM, and the fifth symposium in 2023 on using technology to enhance diversity and inclusivity in neuroscience and neuroimaging. This year, in this sixth DIC symposium, we aim to highlight the importance of promoting diversity, equity and inclusivity in AI techniques for basic and clinical neuroscience. Given the increasing integration of AI and neuroimaging in research and applications, addressing biases and inequalities in these technologies is crucial for their responsible and ethical implementation. This symposium acknowledges and addresses practical challenges in AI for brain research and neuroimaging-based AI models, such as sampling inequalities, biases in predictive models, and the need for transparent and accountable diagnostic classifiers. The symposium aligns with the growing emphasis on ethical AI practices, by highlighting the importance of responsible development and application of AI in brain research to ensure that advancements in technology benefit diverse populations without reinforcing existing disparities.
Objective
- Understanding the impact of population diversity on AI models: (1) Learn about biases in AI models applied to neuroimaging data due to under-representation of diverse populations. (2) Understand how demographic and social determinants influence outcomes of AI models.
- Recognizing sampling inequalities and biases in neuroimaging-based AI models: (1) Understand challenges in neuroimaging-based AI models due to sampling inequalities. (2) Learn about the global and regional variations in sampling inequalities and their impact on model performance. (3) Recognize issues in current diagnostic classifiers and explore strategies for improvement.
- Addressing cross-ethnicity/race generalization failures in neuroimaging based behavioral prediction: (1) Gain awareness of the cross-ethnicity/race generality challenges in this context. (2) Understand consequences of prediction errors and biases against subpopulations. (3) Investigate limitations of training models on specific ethnic groups and bias sources.
Target Audience
Our target audience is the general OHBM membership who are part of and/or attending the OHBM annual meeting.
1. Identifying sources of population covariation in large datasets to protect against model bias
SPEAKER:
Sarah Yip, Yale University New Haven, CT United States
ABSTRACT:
Large scale data collection initiatives, such as the UKB and ABCD study, are poised to provide unprecedented insights into our fundamental understanding of brain development within the context of both health and disease. Given the breadth of ongoing large-scale data collection, both in terms of the number of variables collected and the number of individuals studied, such datasets are further candidates for artificial intelligence (AI) models. However, given the potential for AI models to embed biases arising from under-representation of diverse populations in training data, significant caution should be taken when applying such approaches to neuroimaging and other forms of data. To illustrate this point, we present recent data demonstrating that basic demographic and social determinants of inequity were the primary drivers of day-to-day experiences of hardship during the COVID-19 pandemic. Specifically, using a multivariate pattern-learning approach of >17,000 variables collected from 9,267 families in ABCD to identify baseline predictors of pandemic experiences, as defined by both child and parent report, we find that non-White and/or Spanish speaking families had decreased resources, escalated likelihoods of financial worry and food insecurity. In contrast, those with higher pre-pandemic income and presence of a parent with a postgraduate degree experienced reduced COVID-19 related impact. More recently, we leveraged a deep learning framework (conditional variational autoencoder) in conjunction with the entirety of ABCD behavioral data (n=11875, p=8902) to identify sources of interindividual differences. We find distinct dimensions of diversity driven by factors of socioeconomic status and other environmental factors that can be broadly categorized as social determinants of health. One underlying source of variation reflects material poverty and its health correlates while another captures densely populated living and its disproportionate effects across ethnic groups. Other key stratifications capture privilege via measures of education and income tied to healthy home environments and through European ancestry and desirable neighborhoods in terms of location and air quality. Cognitive ability specifically relating to executive function was related to variation across dimensions. By beginning to untangle the intricate web of such complex associations, we hope that our findings can guide future studies toward relevant covarying diversity measures to be included in brain-behavior modeling efforts when investigating a phenotype of interest. Collectively, these results demonstrate the import of considering basic diversity factors in data-driven analyses of large datasets. Coupled together with other work demonstrating that basic individual difference factors may bias brain-behavior models, they further suggest that, if not explicitly considered, such diversity factors will likely have hidden effects within AI models of neuroimaging data, opening up the potential for significant bias.
- Yip SW, Jordan A, Kohler RJ, Holmes A, and Bzdok D. Multivariate, Transgenerational Associations of the COVID-19 Pandemic Across Minoritized and Marginalized Communities. JAMA psychiatry, 2022. 79(4): 350-358. PMC8829750
- Greene AS, Shen X, Noble S, Horien C, Hahn CA, Arora J, Tokoglu F, Spann MN, Carrión CI, Barron DS, Sanacora G, Srihari VH, Woods SW, Scheinost D, and Constable RT. Brain–phenotype models fail for individuals who defy sample stereotypes. Nature, 2022. 609(7925): 109-118.
2. Sampling inequalities and biases of neuroimaging-based artifact intelligence (AI) models in middle-lower-income countries and areas
SPEAKER:
Zhiyi Chen, Third Military Medical University Experimental Research Center of Medical and Psychological Science Chongqing, AK China
ABSTRACT:
The development of artifact intelligence (AI) models for aiding in the diagnosis of mental disorder is recognized as a significant breakthrough in the field of psychiatry. However, clinical practice of such models remains a challenge, with sampling inequalities and biases being a major limitation. Here, we conducted a pre-registered meta-research assessment on neuroimaging-based models in the psychiatric literature, quantitatively examining global and regional sampling issues over recent decades, from a view that has been relatively underexplored. A total of 476 studies (n = 118,137) were included in the current assessment. Based on these findings, we built a comprehensive 5-star rating system to quantitatively evaluate the quality of existing machine-learning models for psychiatric diagnoses. We also examined risks of bias (ROB) by the structural PROBAST tool. A global sampling inequality in these models was revealed quantitatively (sampling Gini coefficient (G) = 0.81, p < .01), varying across different countries (regions) (e.g., China, G = 0.47; the United State, G = 0.58; Germany, G = 0.78; the United Kingdom, G = 0.87). Further, the severity of this sampling inequality (β = - 2.75, p < .001, R2adj = 0.40; r = - .84, 95% CI: - .41 - -.97) was significantly predicted by national economic levels, and was plausibly predictable for model performance, with higher sampling inequality for reporting higher classification accuracy. Further analyses showed that lack of independent testing (84.24% of models, 95% CI: 81.0-87.5%), improper cross-validation (51.68% of models, 95% CI: 47.2-56.2%), and poor technical transparency (87.8% of models, 95% CI: 84.9-90.8%)/availability (80.88% of models, 95% CI: 77.3-84.4%) are prevailing in current diagnostic classifiers despite improvements over time. In light of this, we proposed a purpose-built quantitative assessment checklist, which demonstrated that the overall ratings of these models increased by publication year but were negatively associated with model performance. Finally, we still found high ROB in predominant existing AI model (83.1%, 80.0 - 86.2%). None of them are perceived to be applicable to clinical practices thus far. Together, improving sampling economic equality and hence the quality of machine-learning models may be a crucial facet to plausibly translating neuroimaging-based diagnostic classifiers into clinical practice.
3. Cross-ethnicity/race generalization failure of RSFC-based behavioral prediction and potential downstream consequences
SPEAKER:
Jingwei Li, Research Center Jülich; Heinrich Heine University Düsseldorf Jülich, Nordrhein-Westfalen Germany
ABSTRACT:
In neuroimaging, a recent, important line of research is to predict behavioral phenotypes from neuroimaging data, e.g. resting-state functional connectivity (RSFC). However, algorithmic unfairness that favors certain subpopulations over others was uncovered in many other machine learning applications but not yet in the application to the neuroimaging field. The risk is high because the predictive models in this field were typically built using large cohorts with mixed ethnic groups, where certain groups, e.g. African Americans (AA), only occupied a very limited proportion. Here, we investigated the cross-ethnicity/race generalizability of the current, field-standard behavioral prediction approach using two large-scale public datasets from the United States: the Human Connectome Project – Young Adults and the Adolescent Brain Cognitive Development cohort. Specifically, prediction errors in AA were much larger than in white Americans (WA) for most behavioral measures. Concerns were raised when looking into the direction of prediction errors. For example, African pre-adolescent participants were more easily overpredicted in social problems, rule-breaking, and aggressive behaviors compared to white participants, leading to a higher false positive rate for AA if such models were directly deployed to diagnose mental disorders. Furthermore, we wondered if training population composition was the main reason for the bias. Therefore, we compared predictive models trained specifically on AA, specifically on WA, or on a mixture of AA and WA with equal sizes. Specific training on AA only helped to slightly reduce the biases against AA, but most of the biases remained. Other possible sources of the biases such as neuroimaging preprocessing (e.g., brain templates and functional atlases) and the design of behavioral measures need to be examined in the future. Our recent follow-up study further discovered a broad association between prediction error amplitudes with all ethnic groups in the datasets beyond the WA-AA comparison, highlighting the severity of this issue.
4. Harnessing population diversity: In search for tools of the trade
SPEAKER:
Jakub Kopal, McGill University Montreal, Quebec Canada
ABSTRACT:
Modern neuroscience is seeing burgeoning population data resources: large-scale datasets with thousands of participant gene expression profiles, brain scanning, and anthropomorphic measures. Such a deep profiling of participants allows us to fully embrace major sources of population diversity – traditionally rarely captured in smaller studies conducted in individual labs. However, big neuroscience datasets are not big small datasets. Emphasis is rebalanced from small, strictly selected, and thus homogenized cohorts towards larger, more representative, and thus diversified cohorts. This shift of context prompts the revision of incumbent modeling practices. In this talk, we will present how predictive tools may fail on new participants due to untracked sources of population diversity. Furthermore, we will present examples of quantitative analytic paradigms and statistical tools that are able to recognize driving factors of population structure, such as ethnicity, height, body composition, gender identity, handedness, language hemisphere dominance, personality, or hormone metabolism. These major sources of population stratification increasingly overshadow the subtle effects that neuroscientists are typically hunting for. That is why dimensions of population stratification need to be treated as effects of interest rather than nuisance variables if the resulting findings are to benefit society as a whole, including marginalized groups. Investing in a new stack of quantitative tools for diversity-aware modeling will bring novel insights into mechanisms behind brain health and disease.